Challenge Summary
Currently, the accessibility and usability of data on the advanced clones and varieties of CIP breeding program available for distribution for breeding and research by external clients are limited, hindering efficient germplasm selection processes. Data on available varieties and advanced clones are not readily accessible or standardized, leading to inefficiencies in analysis and selection by individual breeders. Moreover, the catalog of available germplasm is incomplete and not accessible online for users, exacerbating the challenge of identifying and selecting suitable candidate materials. As a result, researchers, breeders, and farmers face barriers in accessing and evaluating potential germplasm for their breeding programs and agricultural practices.
Hackathon participants are tasked with addressing these challenges by completing the datasets for the potato and sweetpotato catalogues and developing predictive models for efficient germplasm selection. The solution should enable users to access comprehensive and standardized data on available varieties and advanced clones online, facilitating informed decision-making and streamlining the selection process. Furthermore, participants are encouraged to integrate the predictive model with the CIP Genebank request process, enhancing the economy, efficiency and effectiveness of germplasm selection for researchers, breeders, and farmers.
- Utilize natural language processing (NLP) techniques to extract and standardize information from diverse sources, such as research papers, breeding reports, and databases, to complete the datasets for the potato and sweetpotato catalogues.
- Apply machine learning algorithms to identify and resolve inconsistencies or missing data in the catalogues, ensuring accuracy and completeness.
- Develop machine learning models trained on historical breeding data to predict the performance and suitability of different germplasm for specific breeding objectives, considering traits such as yield, disease resistance, and nutritional quality.
- Incorporate geospatial data and environmental factors to enhance the predictive model’s accuracy and robustness, enabling users to identify germplasm adapted to diverse agroecological conditions.
- Implement an application programming interface (API) to seamlessly connect the predictive model with the CIP Genebank request process, allowing users to directly request and access recommended germplasm based on their breeding goals and environmental conditions.
- Utilize reinforcement learning techniques to continuously improve the predictive model’s performance and adaptability based on user feedback and outcomes of germplasm selections.
Crop improvement, commonly referred to as breeding, is a foundational practice in agriculture aimed at developing new crop varieties with desirable traits such as higher yields, resilience to environmental stresses, enhanced nutritional content, and resistance to pests and diseases. Through selective breeding techniques, plant breeders work to combine desired traits from different crop varieties or wild relatives to create superior offspring. These new varieties are rigorously tested and evaluated for performance under various conditions before being released to farmers for cultivation. Crop improvement efforts play a pivotal role in increasing agricultural productivity, ensuring food security, and addressing global challenges such as climate change, poverty, malnutrition, and biodiversity loss. Breeding programs have the potential to yield significant gains across multiple impact areas, including climate adaptability and resilience, poverty alleviation, gender inclusion, biodiversity conservation, and improved nutrition.
By leveraging AI-driven solutions to complete datasets, develop predictive models, and integrate with the CIP Genebank request process, hackathon participants can enhance accessibility, efficiency, and effectiveness in crop germplasm selection, ultimately empowering researchers, breeders, and farmers to make informed decisions and advance agricultural innovation.
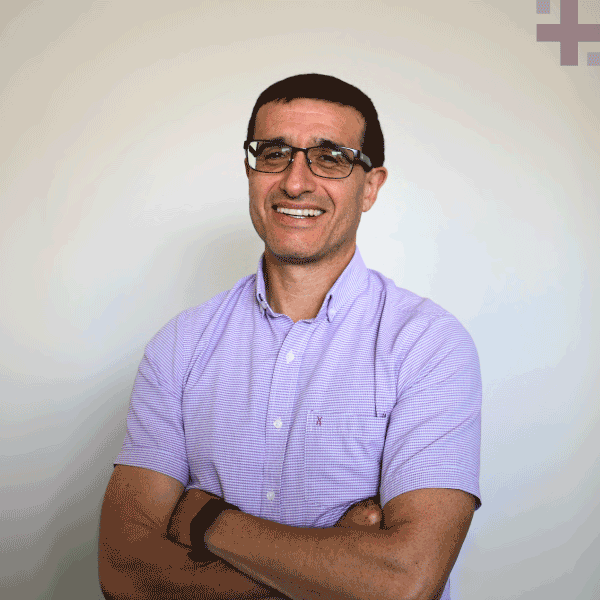