Challenge Summary
Develop predictive models to forecast the winning trait combinations and optimal cultivation zones under different climate change scenarios, considering various disease spread scenarios and evolving consumer demands for Potato, in Peru, Kenya, India or China.
- Utilize machine learning algorithms to analyze historical data on crop traits, yield performance, and consumer preferences to predict optimal trait combinations that align with future market demands.
- Incorporate user-preferred traits from available databases to inform the prediction models.
- Integrate climate data and agroecological factors to identify and map optimal cultivation zones for specific crop varieties under different climate change scenarios.
- Develop geospatial models to assess the suitability of different regions for crop cultivation, considering factors such as temperature, precipitation, soil characteristics, and pest/disease prevalence.
- Account for the potential impacts of climate change on agroecosystems, including shifts in temperature and precipitation patterns, extreme weather events, and changes in pest and disease dynamics.
- Build predictive models to anticipate how climate change may alter disease spread scenarios and incorporate these projections into the optimization of trait combinations and cultivation zones.
- Analyze market trends, demographic shifts, and evolving consumer preferences to forecast future demand for specific crop varieties and traits.
- Develop algorithms to dynamically adjust trait prioritization based on real-time consumer feedback and market dynamics.
Crop improvement, commonly referred to as breeding, is a foundational practice in agriculture aimed at developing new crop varieties with desirable traits such as higher yields, resilience to environmental stresses, enhanced nutritional content, and resistance to pests and diseases. Through selective breeding techniques, plant breeders work to combine desired traits from different crop varieties or wild relatives to create superior offspring. These new varieties are rigorously tested and evaluated for performance under various conditions before being released to farmers for cultivation. Crop improvement efforts play a pivotal role in increasing agricultural productivity, ensuring food security, and addressing global challenges such as climate change, poverty, malnutrition, and biodiversity loss. Breeding programs have the potential to yield significant gains across multiple impact areas, including climate adaptability and resilience, poverty alleviation, gender inclusion, biodiversity conservation, and improved nutrition.
The direct beneficiaries of this solution include breeders at CIP and national research institutes. By leveraging advanced predictive modeling tools and data analytics platforms, breeders can streamline the breeding process, expedite the development of climate-resilient and nutritionally enhanced crop varieties, and enhance collaboration and knowledge sharing, ultimately accelerating the rate of genetic gains and improving the impact of crop improvement initiatives.
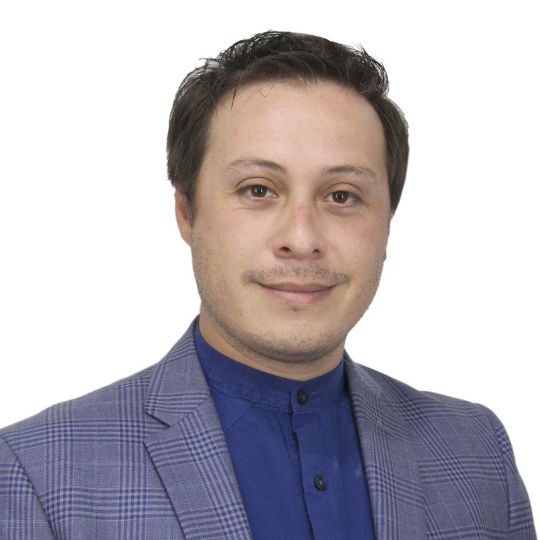
Israel Navarrete
Associate Scientist
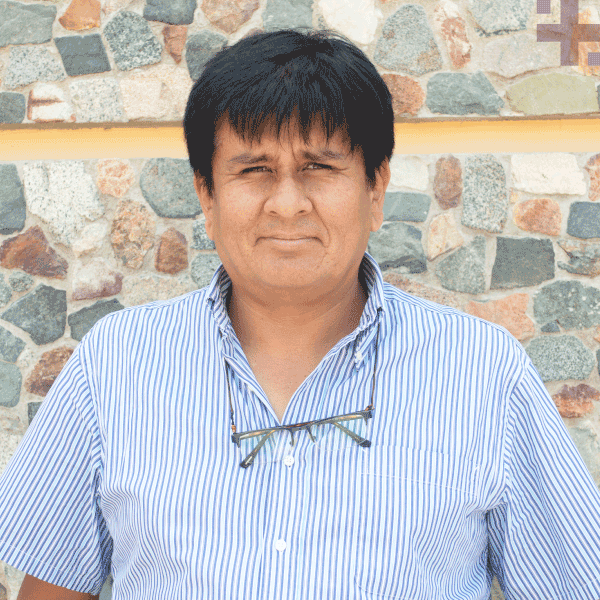
Henry Juarez
Systems and Data Management Officer